The final day of our AI Testing Myths vs. Realities Challenge is upon us! Today, we delve into the dynamic world of mobile AI and its transformative impact on testing.
Mobile technology is at the forefront of innovation, and AI is pivotal in ensuring seamless and effective testing processes. From app performance to user interface consistency, AI is revolutionizing how we approach mobile testing, making it more efficient and reliable.
Let's wrap up our challenge by debunking more AI testing myths and uncovering the realities of mobile AI. Share your thoughts and tell us which one you think is the reality and which is the myth and explain your reasoning in the comments below for a chance to win a ShiftSync gift box, as we conclude this exciting journey.
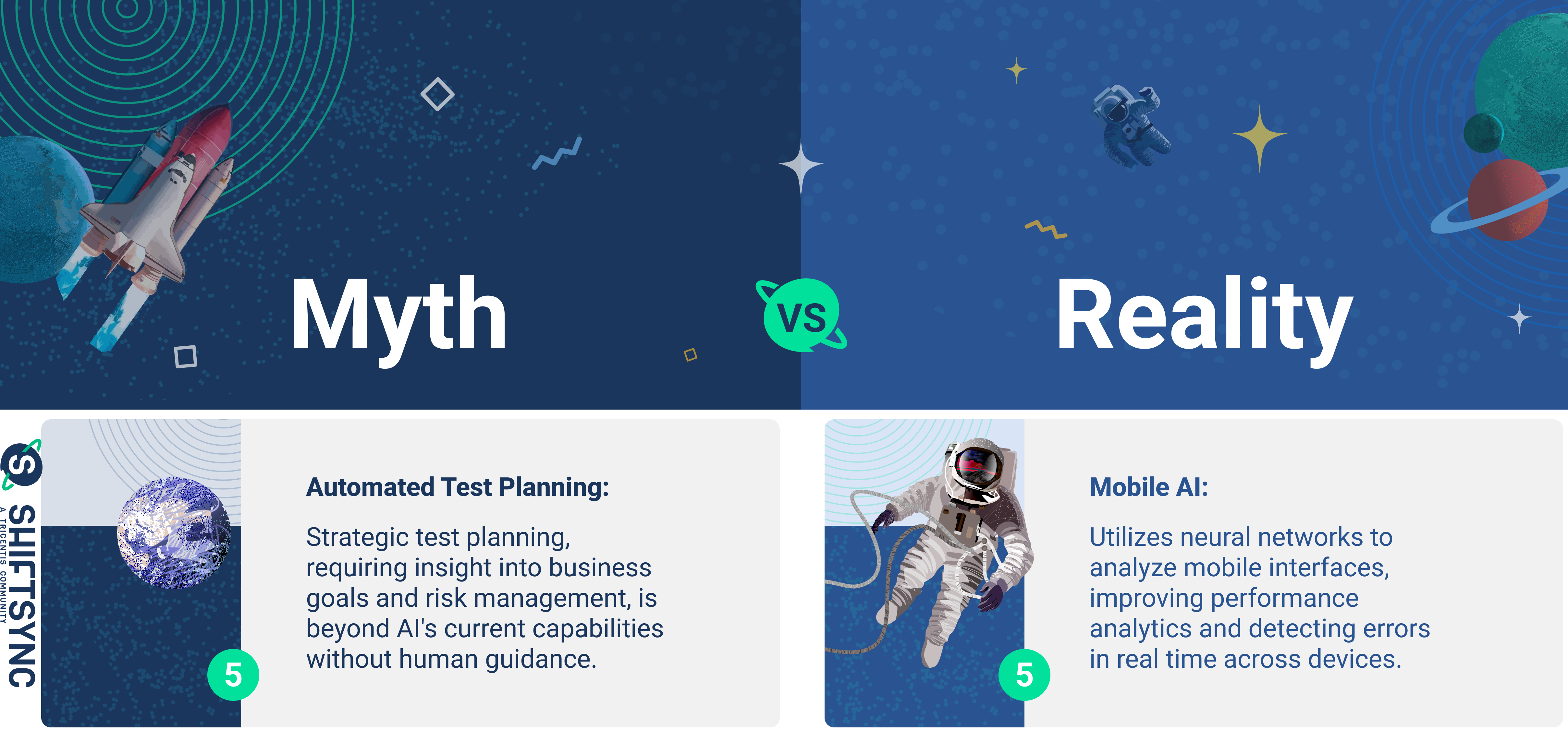
Thank you for being part of our AI Testing Myths vs. Realities Challenge.
Click here to check the rest of the questions.